|
Between land and sea Stefano Guerzoni - Davide Tagliapietra
|
|
 |
|
 |
|
 |
|
 |
|
 |
|
 |
|
|
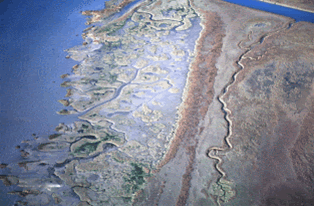 |
|
|
Data processing techniques
We conclude with a technical note, briefly
describing analyses of space-time data and
Geographical Information System (GIS) methods –
regarding point data, type of interpolation, and so
on – used by the authors in producing the maps.
Sometimes symbols with contrasting information
appear to overlap, due to representation of
spatially very close information which is not to
scale.
An important characteristic of the study of the
Lagoon – and of environmental systems in general
– is the involvement of several disciplines (physics,
chemistry, biology, geology, ecology,
environmental sciences, engineering, economics,
archaeology, etc.), whose methods of
measurement and analysis vary widely. These
disciplines produce masses of heterogeneous data,
which enable deeper understanding of the
relations and functions of environmental systems
only if they are interpreted in an integrated and
unified way. Evaluations and predictive models
require integrated analysis of data and
interdisciplinary reading of the processes – only in
this way can management systems and methods
able to support political decision-making be
created.
Complexity and uncertainty exist in all forms of
environmental analysis: from the study of the
distribution and/or evolution of environmental
phenomena to the construction of indices for
evaluating environmental quality. Statistical
methods are a powerful tool in this field, because
they tackle the issue of uncertainty directly and
provide effective techniques for reduction of data
and model-based treatment of complexity.
GISs are an additional tool for analysing territory.
This type of software makes it possible to position
and analyse objects and events which exist and
are verifiable. As an instrument for organising
data relating to land and sea, a GIS enables
researchers to integrate the results of
measurements, models and statistical analyses,
and to generate geographical analyses
accompanied by tables, documents and maps.
Regarding environmental studies, the two main
areas of use are visual representation of patterns
on the geographical level (descriptive use) and
spatial analysis (analytical use). GISs can be of help
in setting up simulation models and, combined
with the use of expert systems, can help to predict
the intensity of pollution or coastal erosion in
various places and in dynamic situations (the
seasonal pattern of chlorophyll, for example).
Visualisation of spatial phenomena is a highly
effective descriptive method. In terms of
environmental analysis and evaluation, a GIS is a
very useful tool for producing thematic maps of
the spatial distribution of various phenomena (for
example, precipitation, surges in river flows,
morphological changes, pollution, nutrients,
salinity, diseases). With access to updated archives,
new maps can be created almost instantly to
represent the current situation, and the spatial
dynamics of the phenomena in question can be
monitored continuously. The level of information is
controlled by the user, who can decide whether to
focus on individual, local cases, or to represent
indices and measurements of frequency, intensity,
prevalence etc., on the level of the geographical,
ecological or administrative unit.
Spatial analysis carried out with GISs includes a
large number of operations. In this field, three
types of spatial data can be analysed: points,
surfaces and geostatistical patterns. The first
problem is the correlation of a small dataset
within a large space and the creation of spatial
variation models. Geostatistical analysis makes it
possible to correlate data from spatially dispersed
points and to determine how they vary in space
and what errors may derive from interpolation
models. The second issue is the evaluation of data
collected at different times, in an environment
which varies widely from year to year. Here too,
statistics can help, by calculating the resulting
errors, thus providing a measure of the reliability
of the analysis.
The maps based on the interpolation of point data
were produced using the Inverse Distance
Weighted method (IDW) as a mathematical
interpolation technique and the ordinary kriging
method as a geostatistical interpolation technique.
IDW predicts the variables in points which were
not directly sampled, assuming that the similarity
of values measured in two points is inversely
proportional to the spatial distance between
them.
Kriging makes it possible to quantify the spatial
autocorrelations between sample points, creating
visualisation surfaces according to the statistical
properties of the distribution of values. Its ability
to compute and assess error and uncertainty helps
to quantify the soundness and reliability of the
method in specific contexts of study. The kriging
method has two precise phases:
1. determination of the spatial structure of the
data;
2. creation of estimates, i.e., prediction of the
interpolated surface.
The former involves calculating the semivariance
(the variation in the similarity of the data with
increasing distance between points of the
dataset). Its values are represented in a
semivariogram, a graph with the distances
between sampled points on the horizontal axis
and the semivariance on the vertical axis. The
resulting semivariogram is independent of the
geographical position of the measurements and
depends only on the distance between them.
The subsequent phase entails the creation of a
model (exponential, circular, gaussian) that seeks
the best approximation of the distribution of the
points in the semivariogram. This is critical for the
whole kriging process, since it directly affects
interpolation and hence data output. The best
approximation of the distribution of the points in
the semivariograms was almost always provided
by the exponential model.
Interpolation by kriging requires the definition of
many parameters so that spatial correlations can
be studied in the best possible way and
approximated with a suitable model. At times,
poor correlation between the measurements of a
dataset may cause intractable problems, with
results that are not always satisfactory.
The maps reproduced here were obtained with
ArcGis 8.3 and 9, using the specific Geostatistical
Analyst extension for processing with the kriging
method.
|
|